Spotify Librarian
Redesign for AI-based music discovery
- Links: demo video
Assignment
For the course DM2630 User Experience Design and Evaluation. The challenge was to “serve music discovery behaviors using AI on new or existing surfaces based on both implicit and explicit signals”.
Role
Lo-fi group design plus hi-fi individual extension. Team: William Rolf, Alexander Widman, William Zhan
The Spotify Librarian is a personalised ape who helps you discover new music. It is inspired by Terry Pratchett’s Discworld, and expands upon the metaphor of “Your Library”.
Ape Intelligence
AI has costs. The design tries to challenge the cornucopian paradigm by being ephemeral, making some of the material costs visible, and possibly making the user reconsider if they really need AI-recommendations right now. The Librarian has a life of their own and doesn’t only exist to please the user.
Music bananas
The explicit signal used is feeding the Librarian with music bananas representing specific songs. This mechanism also couples with the implicit signal – the amount of electricity available on the power grid – which determines how easy or hard it is to pull down and sling the music banana:
Evolving taste and style
Feeding the Librarian affects their recommendations and look. Their taste is ephemeral: after a while their eaten music resets, and the style updates. The idea is to offer a more selective sandbox for music exploration. Librarian-recommended songs appear with the banana icon – in contrast to the (almost ideological) magic sparks:
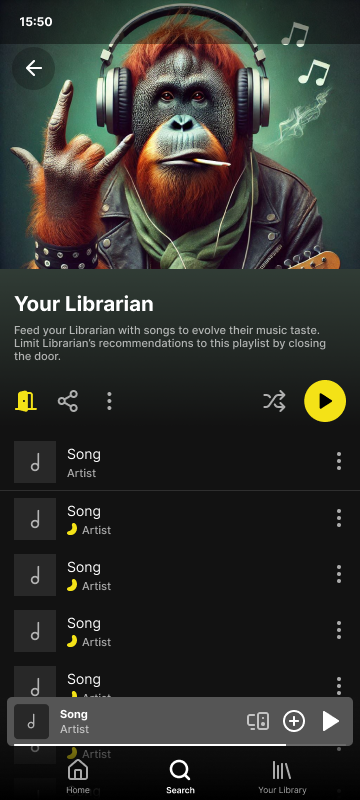
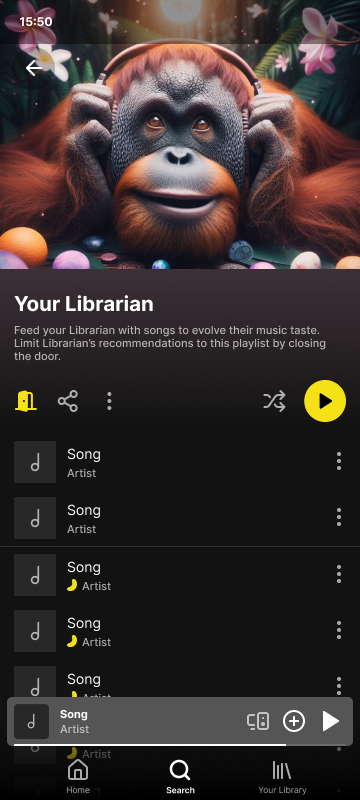
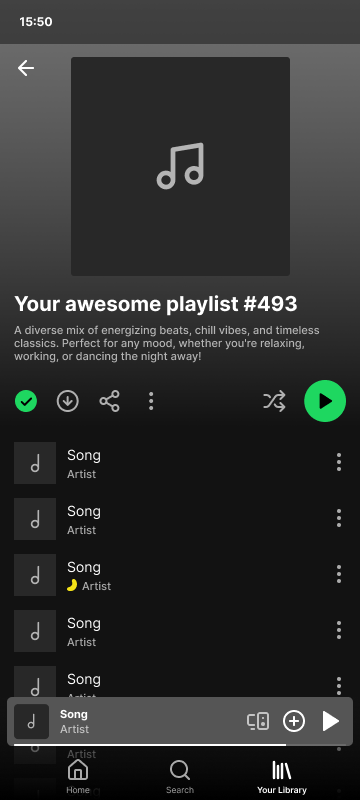
![]() | ![]() | ![]() |
In this prototype they are images generated by DALL-E, but I would prefer something much simpler and cheaper, generating maybe costumes etc. applied to a predefined model.
Hunger
The more hungry the Librarian is, the less precise their recommendations become. If completely empty, they become completely random. This is a way to vary back and forth on the scale of boring–too risky, instead of trying to home in on the “perfect” amount.
Shadow Librarian
If the user instead feeds the Librarian too much, the recommendations instead flip to the opposite of their learned taste. Here I use it to “punish” the user for spending too much electricity, water, etc. – yet it could at the same time help them discover music they never would have otherwise.
This is a playful take on the Waluigi effect: in teaching an AI model “good behavior”, it also implicitly learns “bad behaviour”.
Shadow or random recommendations might be considered a kind of breakdown, putting the user out of auto-pilot mode. Usability is only one of many values we can design for, and here I focussed more on creating a playful experience.
Your Librarian found a friend!
Sometimes the Librarian ventures out into cyberspace and finds friends with similar taste:
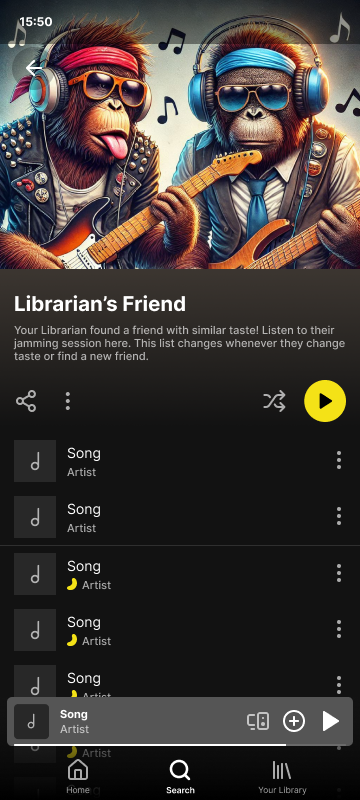
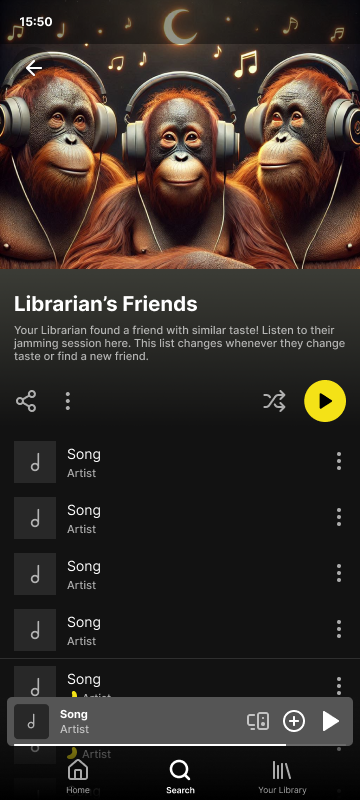
![]() | ![]() |
These are temporary and change when the taste changes, and their users are anonymous. This is to give it a more playful and less serious air, and have a semi-social feature that is consequential.
Process
We started out with mapping how users currently discover new music, and then continued with HMWs and brainstorming:
After settling on and fleshing out an idea, we made a quick low-fi prototype in Miro:
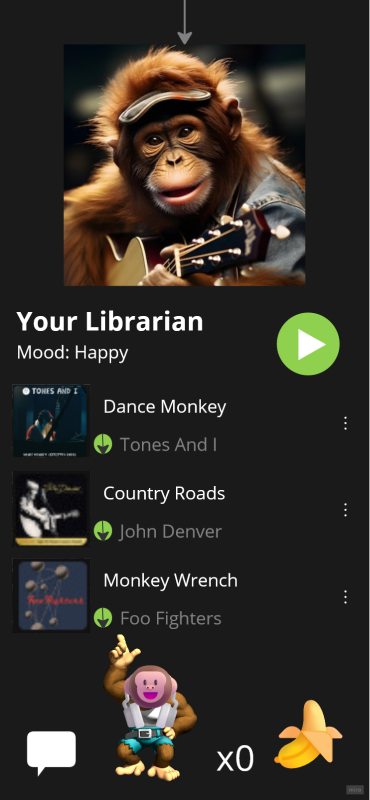
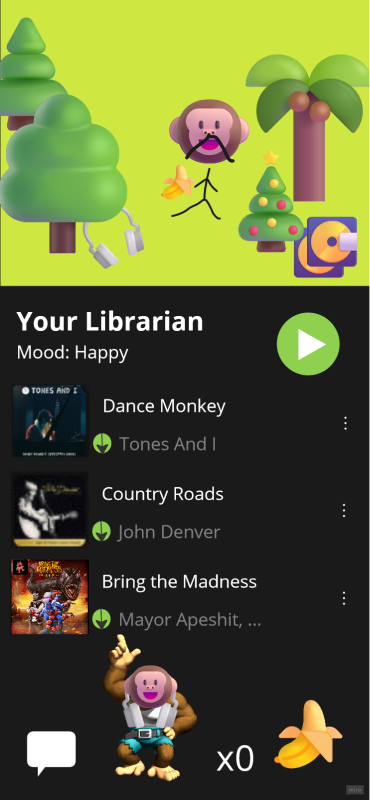
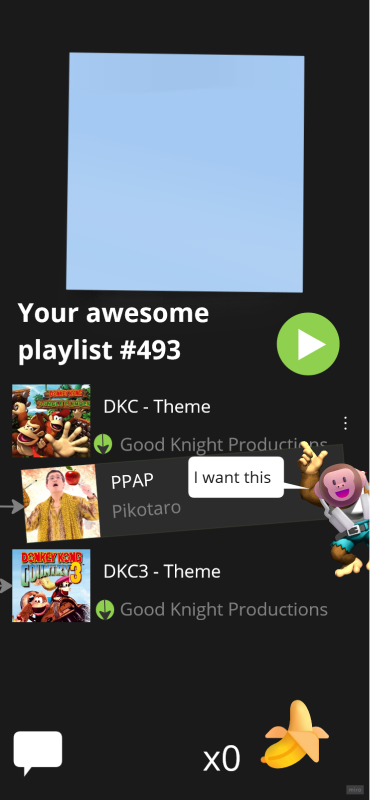
![]() | ![]() | ![]() |
Individually I started by exploring the feeding mechanism – I wanted it to be more unique and playful than a simple button:
Related project: Stamped
Sep – Oct 2023
Assignment
Concept for the course DM2601 Media Technology and Interaction Design. The brief was to design for interdependence.
Team
Kristina Högberg, Edina Niculescu, Lennard Scheibel, Aikaterini Tsioutsia
While the above project was very limited in scope, this course had a focus on the entire design process and its methods. We designed a mobile app fostering social activities and cultural exchange between new and old Stockholmers:
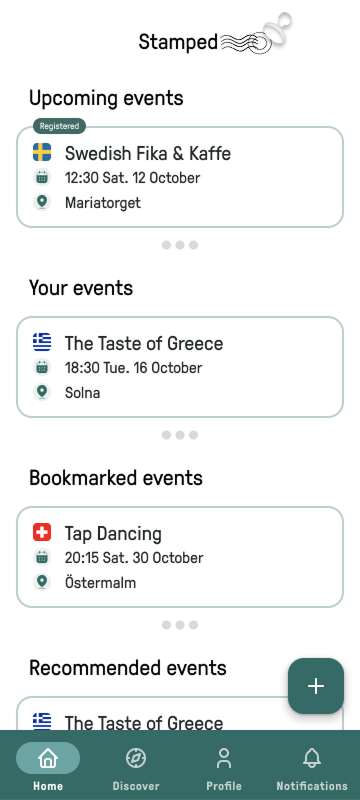
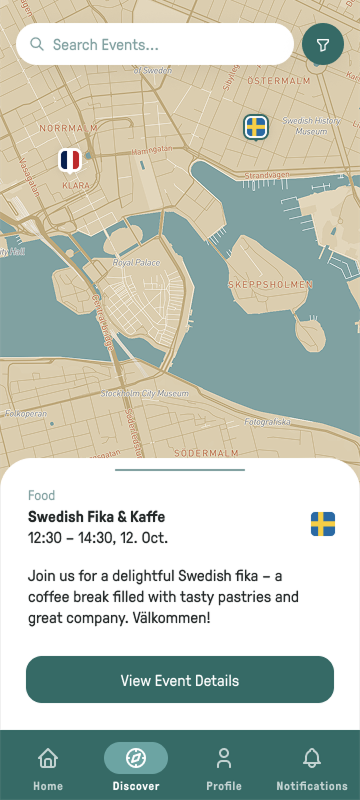
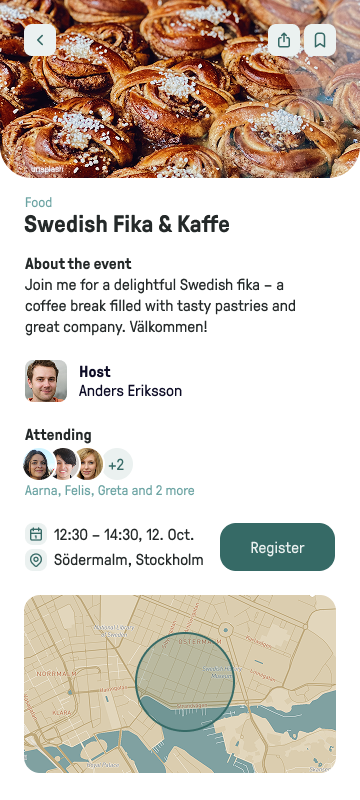
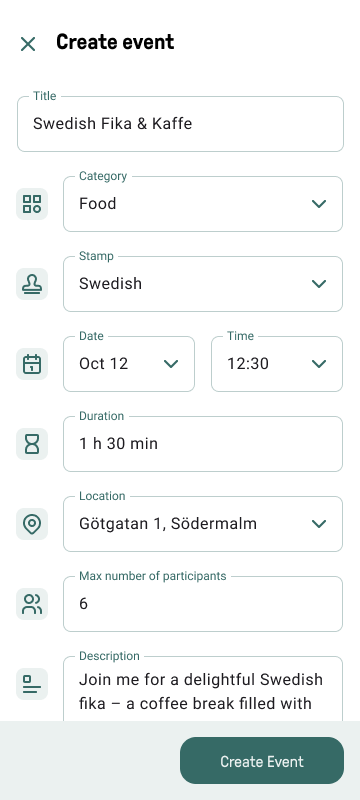
![]() | ![]() | ![]() | ![]() |
We performed interviews, cultural probe, personas, storyboards, affinity diagramming, insights extraction, how might we, brainstorming, brainwriting, parallel design, moodboard, and think-aloud.